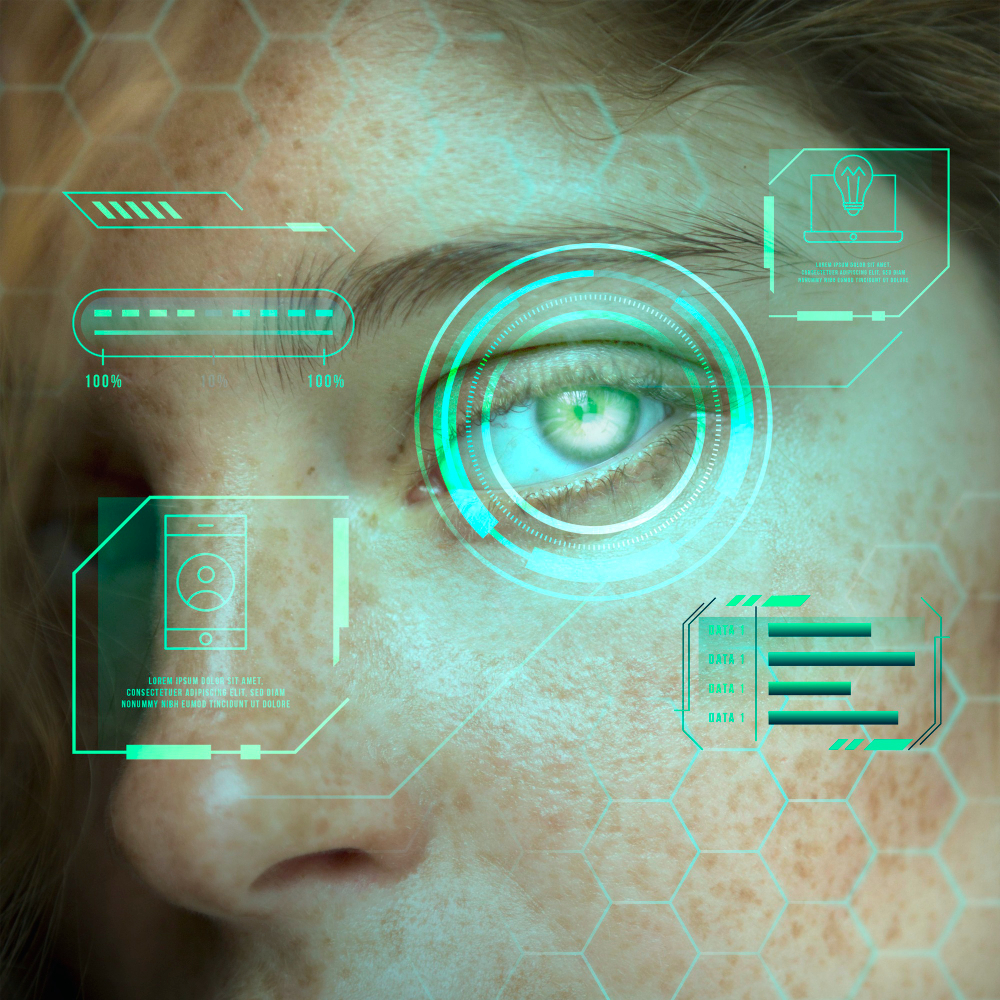
Dana Lane
June 16, 2025
•
15 mins
To achieve precision medicine in mental health, it is crucial to have accurate assessment, monitoring, and feedback data. Precision mental health takes into account not only treatments based on scientific evidence but also the specific needs, preferences, and prognostic potentials of each person. It is essential to integrate data from various biopsychosocial domains to effectively assess an individual's risk and vulnerability (Bickman, Lyon, Wolpert, et al., 2016; Insel, 2015). According to Bickman et al., clinical decision-making within this paradigm should be guided by data. Table 1 provides a summary of seven types of psychological data relevant to mental health treatments and outcomes.
With the integration of digital technologies and data into daily life, a wealth of information beyond self-reported data is available to guide treatment decisions, alleviating the need for extensive measurement. Various types of passively collected data, such as social media activity and physiological measurements, can now be conveniently assessed through mobile phones or wearable devices (Deisenhofer et al., 2024). In this context, the concept of digital phenotype emerged, referring to human digital footprints that hold both diagnostic and prognostic significance (Jain, Powers, Hawkins, & Brownstein, 2015). It should not be confused with the term "digital phenotyping", that denotes the real-time quantification of an individual's phenotype using data from smartphones and other personal digital devices (Tourus, Kiang, Lorme, & Onnela, 2015).
A digital twin (DT) is a virtual representation, in much detail as possible, of a physical entity. They represent an engineering paradigm where physical objects are matched with dynamic digital models. When applied to individuals, DTs leverage detailed bio-physical and lifestyle data to dynamically reflect an individual's molecular and physiological status over time, redefining the notion of 'normality' or 'health' based on individual patterns rather than population averages (Spitzer, Dattner, & Zilcha-Mano, 2023; Bruynseels, Santoni de Sio, & Van den Hoven, 2018)
In the context of digital mental health, DTs can be used to create personalized models of patients, integrating data from various sources such as genetic information, brain imaging, and behavioral patterns. These digital representations can then be used to simulate the effects of different treatments and interventions, allowing clinicians to predict how individuals may respond to specific therapies (Spitzer et al., 2023). The main applications of DTs for health include Hospital Management Design and Care Coordination; Device Design; Biomarker and Drug Discovery; Bio-manufacturing; Surgical Planning; Clinical Trials; Personalized Medicine; and Wellness (Katsoulakis et al., 2024).
Considering a DT as a representation of a patient’s anatomy, physiology, context, history, medical and social conditions, by combining the principles of precision psychiatry with DT technology, clinicians can potentially optimize treatment strategies for individual patients, leading to more effective and personalized care. This integration may ultimately contribute to improved outcomes and a better understanding of mental health disorders (Spitzer et al., 2023; Venkatesh, Brito, Kamel Boulos, 2024). Also, DTs in mental health could be useful for improving the therapist-patient alliance, crucial for therapy success. The Alliance Digital Twin (ADT) could enhance this alliance by providing insights into both trait-like and state-like components of the alliance, aiding therapists in tailoring treatment to individual patient needs. Ultimately, ADT technology aims to improve therapeutic outcomes by strengthening the therapeutic alliance (Spitzer et al., 2023).
In order to be useful, DT has to be individualized, interconnected, interactive, informative, and impactful (Katsoulakis et al., 2024). DTs are not simply one-way maps or shadows of a physical entity; they dynamically represent their physical counterparts. The Health Digital Twin (HDT) integrates general epidemiological data with real-time, patient-specific information for personalized medicine. It harnesses continuous streams of physiological and environmental data from IoT devices like wearables and environmental sensors, along with smartphone apps monitoring physical activity, diet, and mental health. These data, combined with electronic health records (EHR), are processed into clinical markers. Predictive models then generate actionable insights for treatment plans, with feedback loops to refine future predictions. This iterative process ensures the digital twin aligns closely with the physical twin, enhancing accuracy over time (Venkatesh, et al., 2024).
HDTs can help tailor care for patients who do not respond to standard therapies. While clinical guidelines provide general recommendations on dosage, timing, and duration, they often follow a one-size-fits-all model based on clinical trials. HDTs can also aid decision-making in novel situations where real-world evidence is lacking. For instance, when dealing with multiple comorbid or overlapping diseases, HDTs can model the intricate interactions between these conditions and potential treatments, providing valuable insights to guide therapy (Venkatesh, et al., 2024).
HDTs differ from traditional DTs by emphasizing human factors, particularly the user's cognitive mechanisms. While technical implementation has been a focus in literature due to the emerging nature of HDTs, there's a lack of attention to cognitive and psychological considerations. In HDT-based therapy, HDT could support motivation by tracking and visualizing the patient's progress while self-discipline refers to motivation resulting from increased goal orientation through reminders and reward according to the patient's preferences (Lauer-Schmaltz, Cash, Hansen, & Maier, 2022; Gimpel et al., 2013).
DTs involve maintaining comprehensive bio-physical and lifestyle data for individuals over time, redefining 'normality' and 'health' as patterns specific to each person compared to broader population norms. This approach will also influence the distinction between therapy and enhancement. Personalized medicine relies on the idea that advanced mathematical models, powered by extensive biodata, will enable more precise and effective medical interventions. Rather than relying on average responses, digital models promise to customize healthcare based on individual patient predictions. With the growing availability of technologies and computational power, it's becoming feasible to create these personalized models and integrate them with ongoing health and lifestyle tracking, leading to a digital representation of each patient (Bruynseels et al., 2018).
Bickman, L., Lyon, A.R. & Wolpert, M. Achieving Precision Mental Health through Effective Assessment, Monitoring, and Feedback Processes. Adm Policy Ment Health 43, 271–276 (2016). https://doi.org/10.1007/s10488-016-0718-5
Bruynseels, K., Santoni de Sio, F., & Van den Hoven, J. (2018). Digital twins in health care: ethical implications of an emerging engineering paradigm. Frontiers in genetics, 9, 320848. https://doi.org/10.3389/fgene.2018.00031
Chen, I.-M.; Chen, Y.-Y.; Liao, S.-C.; Lin, Y.-H. Development of Digital Biomarkers of Mental Illness via Mobile Apps for Personalized Treatment and Diagnosis. J. Pers. Med. 2022, 12, 936. https://doi.org/10.3390/jpm12060936
Deisenhofer, A.-K., Barkham, M., Beierl, E. T., Schwartz, B., Aafjes-van Doorn, K., Beevers, C. G., Berwian, I. M., Blackwell, S. E., Bockting, C. L., Brakemeier, E.-L., Brown, G., Buckman, J. E. J., Castonguay, L. G., Cusack, C. E., Dalgleish, T., de Jong, K., Delgadillo, J., DeRubeis, R. J., Driessen, E., . . . Cohen, Z. D. (2024). Implementing precision methods in personalizing psychological therapies: Barriers and possible ways forward. Behaviour Research and Therapy, 172, 1–16. https://doi.org/10.1016/j.brat.2023.104443
Gimpel, H., Nißen, M., & Görlitz, R. (2013). Quantifying the quantified self: A study on the motivations of patients to track their own health.
Insel T. Director's Blog: Precision Medicine for Mental Disorders. (2015). Available online at: https://www.nimh.nih.gov/about/directors/thomas-insel/blog/2015/precision-medicine-for-mental-disorders.shtml
Jain, Sachin H., Brian W. Powers, Jared B. Hawkins, and John S. Brownstein. 2015. “The Digital Phenotype.” Nature Biotechnology 33 (5): 462–63. DOI: 10.1038/nbt.3223
Katsoulakis, E., Wang, Q., Wu, H., Shahriyari, L., Fletcher, R., Liu, J., ... & Deng, J. (2024). Digital twins for health: a scoping review. npj Digital Medicine, 7(1), 77. https://doi.org/10.1038/s41746-024-01073-0
Lauer-Schmaltz MW, Cash P, Hansen JP, Maier A. Designing Human Digital Twins for Behaviour-Changing Therapy and Rehabilitation: A Systematic Review. Proceedings of the Design Society. 2022;2:1303-1312. doi: https://doi.org/10.1017/pds.2022.132
Spitzer, M., Dattner, I., & Zilcha-Mano, S. (2023). Digital twins and the future of precision mental health. Frontiers in Psychiatry, 14, 1082598. doi: 10.3389/fpsyt.2023.1082598
Torous, J.; Kiang, M.V.; Lorme, J.; Onnela, J.P. New Tools for New Research in Psychiatry: A Scalable and Customizable Platform to Empower Data Driven Smartphone Research. Jmir Ment. Health 2016, 3, e16. doi: 10.2196/mental.5165
Venkatesh, K. P., Brito, G., & Kamel Boulos, M. N. (2024). Health digital twins in life science and health care innovation. Annual Review of Pharmacology and Toxicology, 64, 159-170. https://doi.org/10.1146/annurev-pharmtox-022123-022046
Recupera tu tranquilidad dedicando solo 5 minutos al día. Fácil, rápido y accesible desde tu móvil. Benefíciate de un seguimiento personalizado y apoyo constante, con objetivos claros que te ayudarán a mantener el rumbo. Un programa práctico y efectivo diseñado para transformarte en tan solo 4 meses.
Tratamientos sin esperas. Programa diario y con apoyo de nuestros terapeutas. Objetivos semanales y duración de 4 meses.
El 89% de nuestros pacientes terminan nuestros programas con grandes mejoras en sus niveles de ansiedad o depresión.
Nuestro enfoque de psicología de precisión permite lograr cambios positivos y duraderos.
Nuestro equipo de psicólogos acreditados ha creado toda una guía para ayudarte con todas tus dudas sobre salud femenina. Somos expertos en cada una de las etapas por las que las mujeres pasamos a lo largo de nuestra vida. Apoyamos a nuestras pacientes para superar la ansiedad, el miedo, el duelo y la pérdida, el bajo estado de ánimo o el estrés.
Te mantendremos informada sobre todo lo que tiene que ver con la tu salud mental.