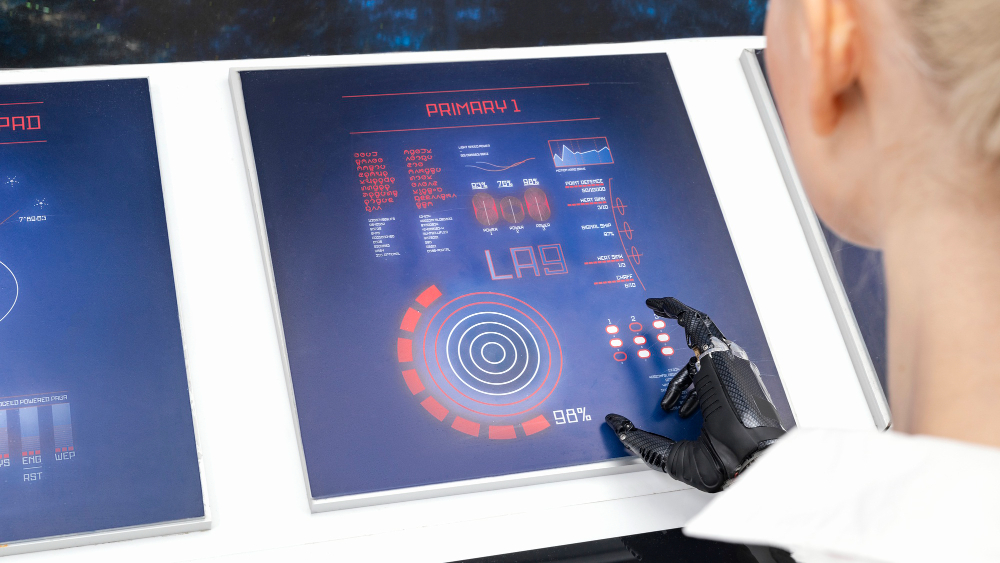
Dana Lane
September 26, 2024
•
15 mins
Precision psychiatry aims to revolutionize mental health care by offering more tailored and efficient treatments through the integration of advanced computational tools, biomarker identification, and data-driven approaches. This article explores the application of precision therapy in mental health, focusing on how technologies such as neuroimaging, ‘omics’ techniques, and wearable devices can improve diagnosis, treatment, and prognosis. By utilizing algorithms, continuous monitoring, and real-time data collection, precision psychiatry seeks to address the heterogeneity of mental disorders, offering patient-specific interventions. Future advancements in precision psychiatry are expected to reshape the landscape of mental health care, focusing on individualized treatment and the interaction between mental and physical health.
La psiquiatría de precisión busca revolucionar el cuidado de la salud mental ofreciendo tratamientos más personalizados y eficientes mediante la integración de herramientas computacionales avanzadas, la identificación de biomarcadores y enfoques basados en datos. Este artículo explora la aplicación de la terapia de precisión en salud mental, centrándose en cómo tecnologías como la neuroimagen, las técnicas 'ómicas' y los dispositivos portátiles pueden mejorar el diagnóstico, tratamiento y pronóstico. Utilizando algoritmos, monitoreo continuo y recopilación de datos en tiempo real, la psiquiatría de precisión aborda la heterogeneidad de los trastornos mentales, proporcionando intervenciones específicas para cada paciente. Se espera que los avances futuros en la psiquiatría de precisión transformen el panorama del cuidado de la salud mental, enfocándose en tratamientos individualizados y la interacción entre la salud mental y física.
In order to improve diagnoses and develop more efficient and tailored treatments, precision psychiatry emerges. Precision psychiatry aims to improve the lives of those with mental illness through accurate diagnosis, prognosis, treatment guidance, and response prediction. Its success would revolutionize mental health care, profoundly reshaping the existing framework of treatment and care (Fernandes, Williams, Steiner, et al., 2017).
Methodologically, the precision approach is rooted in statistical prediction models. In the mid-20th century, doubts emerged about clinicians' predictive abilities compared to statistical methods in diagnosis and treatment determination. Yet, integrating individual clinical assessments with broader statistical data remained a challenge (Van Dellen, 2024).
Precision psychiatry seeks to understand the biological basis of mental illnesses, addressing their complexity and variability among individuals. By integrating data from various sources and employing advanced computational tools, precision psychiatry aims to classify mental illness based on neurobiological factors and develop targeted interventions. It integrates various techniques like 'omics' (genomics, epigenomics, transcriptomics, proteomics, metabolomics, metagenomics and lipidomics), neuroimaging, cognition, and clinical characteristics into domains analyzed using systems biology and computational psychiatry. This process yields biosignatures, sets of biomarkers improving diagnosis, classifications, prognosis, and tailored interventions. Individuals may belong to multiple categories, enabling accurate reclassification, treatment predictions, and prognosis improvements (Fernandes et al., 2017)
Image taken from Fernandes et al 2017.
Van Dellen identifies and summarizes ten challenges in precision psychiatry, proposing different solutions. These include employing data-driven subtyping or prediction modeling to address the lack of a valid gold standard for psychiatric diagnoses, utilizing real-world data or harmonizing naturalistic data to mitigate selection bias in clinical trial data, implementing algorithmic fairness measures and diversity statements to address the lack of diversity and inclusion in precision models, defining minimally effective dose and duration in prediction studies and providing personalized dosing estimates for suboptimal treatment administration. Additionally, strategies such as including estimates of confounding factors in prediction studies, adopting absolute symptom reduction in outcome definition, conducting prospective model validation and implementation studies, and considering contextual factors and employing complex dynamical system approaches to account for the complexity of mental health are suggested to enhance the validity, generalizability, and clinical utility of precision models (Van Dellen, 2024).
Also, precision could be used to improve the assignment of patients to therapists. Constantino et al. (2021) discovered that pairing patients with therapists based on the therapists' strengths in treating particular patient issues resulted in enhanced outcomes compared to standard case assignment practices (Constantino, Boswell, Coyne, Swales, & Kraus, 2021).
To realize precision medicine in mental health, precise assessment, monitoring, and feedback data are essential. Precision mental health considers not only scientifically based treatments but also the unique needs, preferences, and prognostic potentials of each individual. Integration of data encompassing various biopsychosocial aspects is imperative to effectively evaluate an individual's risk and vulnerability (Bickman, Lyon, Wolpert, et al., 2016; Insel, 2015).
Deisenhofer and cols. propose an organizing and conceptual framework called Implementing Precision Methods (IPM) to outline the various components involved in the implementation process. This framework begins with the core element, clinical and practical applications (the first domain), which necessitate the consent and cooperation of clients and clinicians. In precision mental health care, these practical aspects quickly interface with technical considerations (the second domain), such as selecting user-friendly software and hardware. Beyond these technical components, the third domain involves the statistics and analytical procedures critical to precision mental health care, emphasizing the importance of robust algorithms and their continual updates in clinical practice. The outermost domain includes contextual factors (the fourth domain) that impact implementation, such as legal barriers, funding, societal pressures, clinical policies and guidelines, and the setting where implementation occurs (Deisenhofer et al., 2024)
Precision implies the utilization of data and suggests that it should be reserved for methods that are algorithmic, quantitative, and empirically derived. Bringing precision methods into clinical psychology and psychiatry has resulted in data-informed and data-driven psychological therapies. These approaches allow the creation and implementation of decision rules for client treatment, as well as ongoing predictions based on dynamic prediction systems. In such systems, predictions are continuously updated through repeated measurements, often referred to as just-in-time adaptive interventions. Additionally, precision may involve automatic adaptation of digital therapy to meet a patient's needs based on collected data (Deisenhofer, et al., 2024).
Precision mental health offers a chance for the discipline to progress beyond conventional self-reported information (Torous and Baker, 2016). At the heart of the m-Health phenomenon is the global rise in smartphone usage and the development of cost-effective wearables offering advanced features, improved connectivity, and precise monitoring of behavioral and physiological data (Bidargaddi, Schrader, Klasnja, Licinio and Murphy, 2020).
Precision medicine has brought numerous benefits when applied to groups, but the next significant change will occur when we stop comparing people to each other and instead focus on comparing each person to themselves. Embracing individualized continuous monitoring through consumer wearable devices offers an interesting future direction for precision psychiatry. This approach can help us better understand the dynamic interactions between mental health and physiological states. By improving our understanding of mental health at a physiological level, precision medicine can help bridge the gap between different medical specialties and highlight the connection between mental disorders and biological systems (Scala, Ganz, & Snyder, 2023).
The underlying mechanisms of psychiatric disorders have identified biomarkers that may indicate early signs of mental health risks. This exploration has led to the identification of psychiatric biomarkers reflecting anomalies in different systems. Recent advancements in biosensors have facilitated the detection of key psychiatric biomarkers like cortisol, dopamine, and serotonin, employing different biofluid platforms and sensing principles. However, to enhance accessibility, these biosensors need integration with portable, wearable, or microfluidic devices, and bringing mental health care closer to individuals. Moreover, synchronizing daily data on psychiatric indicators, clinical cases, and experiences globally can establish a comprehensive 'Big-Data' network, fostering advancements in mental healthcare prevention, diagnosis, treatment, and prognosis (Wang, Hu, Jiang, & Yetisen, 2024).
Over the past decade, wearable systems for monitoring biological signals have evolved significantly, driven by advancements in flexible electronics, energy storage, wireless data transmission, and information processing technologies. These sensors track mental status through physiological activities, physical movements, and biochemical profiles. Wearable sensors offer promising potential as objective and continuous monitoring tools for mental health, replacing subjective and time-consuming methods like interviews. These sensors detect various body responses to stressors, including changes in cardiac activity, respiration, sweat secretion, and stress hormone levels (Kang & Chai, 2022)
A review conducted by Dobson and cols. explored the effectiveness of wearable sensors for detecting, managing, and treating anxiety.Their findings showcased notable improvements in anxiety and stress levels, alongside the integration of coping strategies like diaphragmatic breathing. Moreover, the sensors demonstrated the capability to offer interventions, such as guiding users through relaxation exercises, thus extending their utility beyond mere detection. The review further highlighted the diverse applicability of sensors across different populations and contexts, ranging from mild stress levels to diagnosed clinical disorders (Dobson, Li, Garner, Tane, McCool, & Whittaker, 2023). Similarly, meta-analysis conducted by Čukić and cols. revealed that Heart rate variability (HRV) in depression had an overall effect size of 1.03 (95% CI 0.703-1.35) for the connection between nonlinear HRV measures and depression. Subgroup analyses showed significant effectiveness for detrended fluctuation analysis (ES 0.364, 95% CI 0.237-0.491), entropy-based measures (ES 1.05, 95% CI 0.572-1.52), and other nonlinear measures (ES 0.702, 95% CI 0.422-0.982). The results suggest HRV potential for early screening of cardiovascular abnormalities in depressed patients to mitigate adverse events (Čukić, Savić, & Sidorova, 2023).
A recent review focuses on the feasibility and effectiveness of using smartphones to track insomnia, depression, and anxiety in adults and young adults. The authors highlighted that using smartphone sensors to identify insomnia could enable early detection or intervention for mental health issues like depression and anxiety (Alamoudi, Breeze, Crawley, & Nabney, 2023). Other studies also found that measures of activity and sleep from smartphone activity were strongly related to and somewhat predictive of participants’ well-being. This reaffirms the importance of considering physical activity and sleep for predicting mood and making automatic mood monitoring a reality (DeMasi, Feygin, Dembo, Aguilera, & Recht, 2017)
Smartphones constitute rich sources of digital data that reflect users' emotional states, behaviors, and cognitive patterns. These encompass diverse elements such as microphones, motion sensors, interactions with digital interfaces, and screen usage. For instance, speech analysis can decipher a user's emotional state through variations in volume, tone, and language choice. Similarly, motion sensors track spatial movements and activity levels, shedding light on users' daily routines and social interactions. These digital biomarkers offer a comprehensive picture of individuals' mental well-being and activities in real time (Chen, Chen, & Lin, 2022). Understanding digital phenotyping involves examining the data collected from smartphone sensors and usage patterns. Initially, the range and types of data generated by smartphone sensors and usage patterns may seem overwhelming, yet they offer valuable insights into various social and behavioral aspects of mental health conditions (Onnela, Rauch, Harnessing, 2016).
As Chen and cols. explain, apps like Know Addiction or Menthal for Android and Screen Time for iOS exemplify mobile applications that convert screen activities into digital biomarkers. Typically, these apps gather three main types of data: timestamps indicating screen on/off events, timestamps of received notifications, and details about the apps accessed. Subsequently, this data is analyzed to generate a digital profile, enabling the interpretation of behavioral tendencies and the prediction of user engagement (Chen and cols. 2022).
Historically, mental health care models have been reactive, but emerging approaches prioritize early screening and continuous monitoring through digital phenotyping. This method enables early detection of potential risks, facilitating timely intervention to prevent crises. Digital phenotyping provides objective insights into various aspects of mental health, allowing for the identification of subtle changes that may indicate relapse or crisis, such as alterations in speech patterns or social behavior (Onnela et al., 2016). This could be particularly useful in suicidal ideation (Diniz et al., 2022).
The term "precision" implies the use of data and suggests that it should be reserved for methods that are algorithmic, quantitative, and empirically derived. Applying precision techniques to clinical psychology and psychiatry has resulted in data-informed and data-driven psychological therapies, where decision rules for client treatment can be established and implemented from the start. Additionally, during ongoing psychological treatment, it involves making predictions based on dynamic prediction systems, which are constantly updated through the collection of new data via repeated measurements, often referred to as just-in-time adaptive interventions. Precision can also mean that digital therapies are automatically tailored to a patient's needs based on data (Deisenhofer et al., 2024).
Applied to psychological disorders, the precision approach addresses the issue that, despite recognizing depression's heterogeneity, treatments remain largely uniform across different clinical profiles. This uniformity could explain why only about 30% of patients recover with their first medication and 55% experience side effects. The precision approach proposes tailoring treatments based on depressive subtypes and patient-specific factors like personality and comorbidities, rather than using a one-size-fits-all method (Deif & Salama, 2021).
Numerous precision psychiatry interventions are currently undergoing testing in clinical trial settings. The StratCare Trial assessed the effectiveness of a machine learning algorithm in aiding treatment selection for depression, anxiety, and post-traumatic stress disorder in a cohort of 951 patients. The algorithm incorporated clinical variables, such as patient-reported levels of depression, anxiety, and functional impairment, alongside demographic data like employment status, race, and ethnicity. With this information, the algorithm assisted clinicians in implementing stratified care by suggesting either low- or high-intensity treatment options. Patients in the algorithm-assisted group were 7% more likely to see a reduction in depressive symptoms and required fewer treatment sessions, at an additional cost of $150 per patient. Key benefits of the StratCare approach include its demonstrated, albeit modest, clinical effectiveness, applicability across multiple disorders, and economic viability (Delgadillo, et al., 2022).
A prospective randomized-controlled trial explored the effects of a digital decision support and feedback system offering patient-specific recommendations, including clinical strategy suggestions and adaptive advice for patients at risk of treatment failure. The findings indicated that following treatment strategy recommendations for the first 10 sessions led to a modest improvement in outcomes. Psychometric feedback enhanced with clinical problem-solving tools also positively impacted treatment results. Although feedback alone did not show main effects, the study underscores the importance of high-quality implementation of digital decision support tools. This trial provides valuable insights into the use of digital decision support systems in mental health, highlighting the potential for such tools to improve psychotherapy practices and the critical role of proper implementation for their success (Lutz, Deisenhofer, Rubel, Bennemann, Giesemann, Poster, & Schwartz, 2022).
Despite significant advancements in precision psychiatry through computational modeling, genomics, and biomarker discovery, these innovations have yet to become standard clinical practice, limiting their benefits to the average patient. While promising, current research often lacks diverse representation and real-world applicability, with many studies not validated on independent cohorts. Future progress in precision psychiatry should focus on integrating real-world data to develop and test tools within clinical settings, addressing biases and small sample sizes. As precision medicine evolves, shifting towards individual-specific comparisons and continuous monitoring with wearable devices could provide deeper insights into the interplay between mental health and physiological states, ultimately enhancing treatment across various medical specialties and improving overall patient care. Precision mental health care is not only beneficial for psychiatric patients but also crucial across various medical specialties such as endocrinology, cardiology, oncology, gastroenterology, and pain management. The World Health Organization projects that mental illness will be the leading cause of morbidity by 2030, highlighting its profound impact on psychological, biological, and lifestyle factors. Given that mental illness significantly contributes to major health issues and leading causes of death, precision psychiatry offers a promising approach to better understand its molecular basis and provide more effective, comprehensive care that addresses both mental and physical health needs (Scala, Ganz, & Snyder, 2023).
References
Alamoudi, D., Breeze, E., Crawley, E., & Nabney, I. (2023). The feasibility of using smartphone sensors to track insomnia, depression, and anxiety in adults and young adults: narrative review. JMIR mHealth and uHealth, 11, e44123. doi: 10.2196/44123
Bidargaddi N, Schrader G, Klasnja P, Licinio J, Murphy S. Designing m-Health interventions for precision mental health support. Transl Psychiatry. 2020 Jul 7;10(1):222. doi: 10.1038/s41398-020-00895-2. PMID: 32636358; PMCID: PMC7341865.
Chen, I. M., Chen, Y. Y., Liao, S. C., & Lin, Y. H. (2022). Development of digital biomarkers of mental illness via mobile apps for personalized treatment and diagnosis. Journal of Personalized Medicine, 12(6), 936. https://doi.org/10.3390/jpm12060936
Constantino, M. J., Boswell, J. F., Coyne, A. E., Swales, T. P., & Kraus, D. R. (2021). Effect of matching therapists to patients vs assignment as usual on adult psychotherapy outcomes: A randomized clinical trial. JAMA Psychiatry, 78(9), 960-969. doi: 10.1001/jamapsychiatry.2021.1221
Čukić, M., Savić, D., & Sidorova, J. (2023). When heart beats differently in depression: review of nonlinear heart rate variability measures. JMIR Mental Health, 10(1), e40342. doi: 10.2196/40342
Deif R, Salama M. Depression From a Precision Mental Health Perspective: Utilizing Personalized Conceptualizations to Guide Personalized Treatments. Front Psychiatry. 2021 May 11;12:650318. doi: 10.3389/fpsyt.2021.650318. PMID: 34045980; PMCID: PMC8144285.
Deisenhofer, A. K., Barkham, M., Beierl, E. T., Schwartz, B., Aafjes-van Doorn, K., Beevers, C. G., & Cohen, Z. D. (2024). Implementing precision methods in personalizing psychological therapies: Barriers and possible ways forward. Behaviour research and therapy, 172, 104443. https://doi.org/10.1016/j.brat.2023.104443
Delgadillo, J., Ali, S., Fleck, K., Agnew, C., Southgate, A., Parkhouse, L., ... & Barkham, M. (2022). Stratified care vs stepped care for depression: A cluster randomized clinical trial. JAMA psychiatry, 79(2), 101-108. https://doi.org/10.1001/jamapsychiatry.2021.3539
DeMasi, O., Feygin, S., Dembo, A., Aguilera, A., & Recht, B. (2017). Well-being tracking via smartphone-measured activity and sleep: cohort study. JMIR mHealth and uHealth, 5(10), e7820. doi: https://doi.org/10.2196/mhealth.7820
Diniz, E.J.S.; Fontenele, J.E.; de Oliveira, A.C.; Bastos, V.H.; Teixeira, S.; Rabêlo, R.L.; Calçada, D.B.; dos Santos, R.M.; de Oliveira, A.K.; Teles, A.S. Boamente: A Natural Language Processing-Based Digital Phenotyping Tool for Smart Monitoring of Suicidal Ideation. Healthcare 2022, 10, 698. https://doi.org/10.3390/healthcare10040698
Dobson R, Li LL, Garner K, Tane T, McCool J, Whittaker R The Use of Sensors to Detect Anxiety for In-the-Moment Intervention: Scoping Review JMIR Ment Health 2023;10:e42611 doi: 10.2196/42611
Fernandes, B.S., Williams, L.M., Steiner, J. et al. (2017). The new field of ‘precision psychiatry’. BMC Medicine, 15, 80. https://doi.org/10.1186/s12916-017-0849-x
Insel, T. (2015). Director's Blog: Precision Medicine for Mental Disorders. Available online at: https://www.nimh.nih.gov/about/directors/thomas-insel/blog/2015/precision-medicine-for-mental-disorders.shtml
Kang, M.; Chai, K. Wearable Sensing Systems for Monitoring Mental Health. Sensors 2022, 22, 994. https://doi.org/10.3390/s22030994
Lutz, W., Deisenhofer, A. K., Rubel, J., Bennemann, B., Giesemann, J., Poster, K., & Schwartz, B. (2022). Prospective evaluation of a clinical decision support system in psychological therapy. Journal of consulting and clinical psychology, 90(1), 90. https://doi.org/10.1037/ccp0000642
Onnela, JP., Rauch, S. Harnessing Smartphone-Based Digital Phenotyping to Enhance Behavioral and Mental Health. Neuropsychopharmacol 41, 1691–1696 (2016). https://doi.org/10.1038/npp.2016.7
Scala, J. J., Ganz, A. B., & Snyder, M. P. (2023). Precision medicine approaches to mental health care. Physiology, 38(2), 82-98. https://doi.org/10.1152/physiol.00013.2022
Torous, J., & Baker, J. T. (2016). Why psychiatry needs data science and data science needs psychiatry: connecting with technology. JAMA psychiatry, 73(1), 3-4.DOI: 10.1001/jamapsychiatry.2015.2622
van Dellen, E. (2024). Precision psychiatry: predicting predictability. Psychological Medicine. Published online 2024:1-10. https://doi.org/10.1017/S0033291724000370
Wang, L., Hu, Y., Jiang, N., & Yetisen, A. K. (2024). Biosensors for psychiatric biomarkers in mental health monitoring. Biosensors and Bioelectronics, 116242. https://doi.org/10.1016/j.bios.2024.116242
Recupera la calma con sólo 5 minutos al día.
Fácil y rápido desde tu móvil. Seguimiento y apoyo diario con objetivos claros. Programa corto de 4 meses.
Tratamientos sin esperas. Programa diario y con apoyo de nuestros terapeutas. Objetivos semanales y duración de 4 meses.
El 89% de nuestros pacientes terminan nuestros programas con grandes mejoras en sus niveles de ansiedad o depresión.
Nuestro enfoque de psicología de precisión permite lograr cambios positivos y duraderos.
Nuestro equipo de psicólogos acreditados ha creado toda una guía para ayudarte con todas tus dudas sobre salud femenina. Somos expertos en cada una de las etapas por las que las mujeres pasamos a lo largo de nuestra vida. Apoyamos a nuestras pacientes para superar la ansiedad, el miedo, el duelo y la pérdida, el bajo estado de ánimo o el estrés.
Te mantendremos informada sobre todo lo que tiene que ver con la tu salud mental.